Breaking Records in Generative AI: NVIDIA Dominates MLPerf Training v4.0
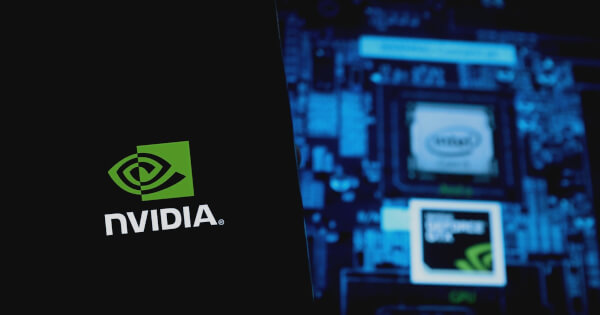
NVIDIA, a powerhouse in the tech industry, has once again proven its dominance in the realm of generative AI. Their recent submission to MLPerf Training v4.0 has set new performance and scale records, solidifying their position as a leader in AI training benchmarks, especially in large language models (LLMs) and generative AI.
MLPerf Training v4.0 Updates
MLPerf Training, created by the MLCommons consortium, is the gold standard for evaluating AI training performance. The latest iteration, v4.0, includes new tests that reflect popular industry workloads. These tests cover a range of tasks, including fine-tuning speed of Llama 2 70B with LoRA technique and graph neural network (GNN) training using relational graph attention network (RGAT) implementation.
In addition to these tests, the updated suite includes workloads like LLM pre-training (GPT-3 175B), text-to-image (Stable Diffusion v2), and more, offering a comprehensive evaluation of AI capabilities.
NVIDIA’s Record-Breaking Performance
Using their cutting-edge hardware and software solutions, NVIDIA has achieved remarkable performance milestones in the latest MLPerf Training round. The combination of NVIDIA Hopper GPUs, NVLink interconnect, Quantum-2 InfiniBand networking, and optimized software stack has catapulted NVIDIA to new heights. Notably, their GPT-3 175B training time has been significantly reduced, showcasing impressive scalability and efficiency.
Advancements in Generative AI
NVIDIA’s breakthroughs in LLM fine-tuning with the Llama 2 70B model and advancements in text-to-image generative AI illustrate their commitment to pushing boundaries in AI research. Leveraging tools like the NVIDIA NeMo framework and FP8 implementation of self-attention in cuDNN, NVIDIA has unlocked new levels of performance and efficiency in generative AI tasks.
Graph Neural Network Training
In addition to their successes in generative AI, NVIDIA has also achieved record times in GNN training. By utilizing their powerful GPUs, NVIDIA has demonstrated exceptional speed and accuracy in training large-scale GNN models, further solidifying their position as a frontrunner in AI research and development.
Key Takeaways
NVIDIA’s continuous innovations in AI training performance underscore their commitment to advancing the field. By optimizing their software stack and exploring new frontiers in AI research, NVIDIA is paving the way for more efficient and cost-effective AI training. The future looks promising with the upcoming NVIDIA Blackwell platform, promising faster real-time trillion-parameter inference and training, setting the stage for groundbreaking AI applications.
For a deeper dive into NVIDIA’s achievements, visit the NVIDIA Technical Blog for detailed insights and updates.
Image source: Shutterstock