Welcome to Extreme Investor Network
At Extreme Investor Network, we bring you the latest and most in-depth information on all things crypto, cryptocurrency, blockchain, and more. Our team of experts is dedicated to providing you with unique insights and valuable content that you won’t find anywhere else. Today, we are excited to discuss the groundbreaking developments in computational fluid dynamics (CFD) brought about by NVIDIA Modulus and machine learning.
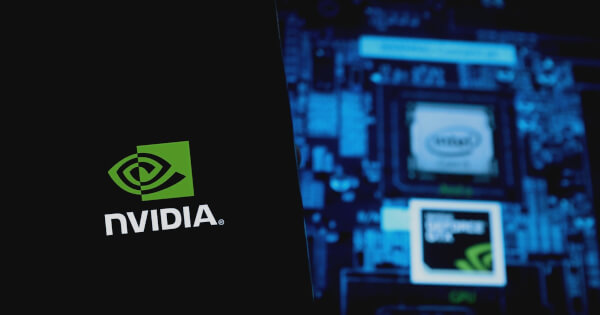
In a game-changing move, NVIDIA Modulus is revolutionizing the field of computational fluid dynamics by incorporating machine learning techniques. This integration addresses the challenges associated with high-fidelity fluid simulations, offering improved computational efficiency and accuracy for complex flow modeling.
Unlocking the Power of Machine Learning in CFD
Machine learning, specifically through Fourier neural operators (FNOs), is reshaping CFD by reducing computational costs and enhancing model precision. FNOs enable the training of models on lower-resolution data that can be seamlessly integrated into high-fidelity simulations, resulting in significant cost savings.
NVIDIA Modulus serves as an open-source platform that supports the implementation of FNOs and other advanced ML models. With optimized algorithms, Modulus becomes a versatile tool for various applications in the field of computational fluid dynamics.
Leading Research at Technical University of Munich
The Technical University of Munich, under the leadership of Professor Dr. Nikolaus A. Adams, is at the forefront of incorporating ML models into traditional simulation workflows. Their innovative approach combines the accuracy of numerical methods with the predictive capabilities of artificial intelligence, leading to substantial performance enhancements.
By integrating ML algorithms like FNOs into the lattice Boltzmann method (LBM) framework, the TUM team has achieved remarkable speedups compared to conventional CFD methods. This hybrid approach is revolutionizing the efficiency of solving complex fluid dynamics problems.
Hybrid Simulation Environment and Future Outlook
The TUM team has developed a hybrid simulation environment that integrates ML into the LBM framework, enabling efficient computation of multiphase and multicomponent flows in intricate geometries. By leveraging PyTorch for implementing LBM, the team has created the fast and user-friendly TorchLBM solver, resulting in significant computational efficiency gains.
Looking ahead, the TUM team plans to further enhance their hybrid models, scale simulations with multi-GPU setups, and integrate workflows into NVIDIA Omniverse. These advancements set a new benchmark in CFD research, showcasing the transformative potential of machine learning in fluid dynamics.
As more researchers adopt similar methodologies, industries are poised to benefit from more efficient designs, improved performance, and accelerated innovation. NVIDIA’s commitment to providing advanced AI tools through platforms like Modulus continues to advance this transformation.
Stay tuned to Extreme Investor Network for more exclusive insights and updates on the latest trends in the world of crypto, cryptocurrency, blockchain, and beyond.