Welcome to Extreme Investor Network!
As experts in the world of cryptocurrency and blockchain technology, we are excited to bring you the latest insights and information on cutting-edge developments in the industry. Today, we are diving into the innovative world of Direct Preference Optimization (DPO) with synthetic data, as explored by Anyscale in their latest blog post.
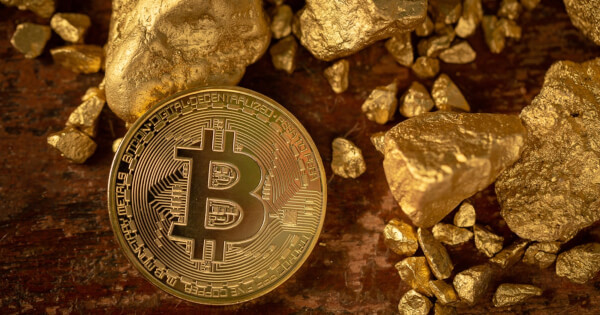
Anyscale’s exploration of Direct Preference Optimization (DPO) sheds light on a significant methodology for tuning language models to better align their outputs with human preferences. In their latest blog post, Anyscale provides a detailed case study on the application of DPO using synthetic data, specifically focusing on summarization tasks.
The Power of Synthetic Data Generation
Synthetic data generation has revolutionized the process of creating high-quality datasets. Anyscale’s approach leverages AI models as data augmenters and judges, leading to improvements in subsequent models. Their blog outlines a comprehensive pipeline for synthetic data generation, highlighting the efficacy of Ray Data and vLLM for scaling and rapid experimentation.
Unlocking Insights through DPO Training
Direct Preference Optimization (DPO) strikes a balance between complexity and effectiveness, making it a widely adopted algorithm for preference tuning. Anyscale has seamlessly integrated DPO into its LLM suite, empowering users to craft preference-tuned models through an intuitive API. The blog delves into key modeling insights and experiments conducted on DPO for summarization tasks.
Crucial Evaluation Techniques
Anyscale relies on Ray Data and vLLM for batch inference to evaluate generated summaries at scale. Evaluation serves as a critical benchmark for assessing model quality, with Anyscale emphasizing the need for task-specific evaluation aligned with training objectives. The blog provides essential details on setting up preference functions for effective evaluation.
Comparing DPO with Traditional Approaches
The blog presents a compelling comparison between DPO and traditional supervised fine-tuning (SFT) methods. While SFT requires high-quality data collection and exact imitation of desired behavior, preference tuning focuses on determining whether one response is preferred over another. This approach enables scalable data generation and on-policy data collection, effectively addressing model-specific challenges.
Case Study: Enhancing Summarization with DPO
Anyscale implements DPO in the Mistral-7B-instruct-v0.1 model for summarizing CNN articles in a groundbreaking case study. By designing a synthetic summarization preference dataset and leveraging a synthetic judge, Anyscale minimizes costs and ensures alignment between training and evaluation. The preference function combines word count minimization and Q&A accuracy for evaluating summaries.
Key Insights from Data Generation and DPO Training
Anyscale leverages the Mistral-7B-Instruct-v0.1 model for on-policy data generation and implements DPO within its LLM post-training offering. Through detailed examples of DPO training configurations and focus on critical hyperparameters, Anyscale showcases the power of efficient training using Ray.
Driving Performance through Iterative On-Policy Training
Anyscale emphasizes iterative on-policy training as a strategy to enhance DPO performance, leading to significant performance gains. By regenerating training data with fine-tuned models and applying additional DPO rounds, Anyscale achieves competitive performance comparable to traditional RLHF methods.
For a detailed case study and methodology, we highly recommend referring to the original post on Anyscale. Stay tuned to Extreme Investor Network for more exclusive insights and updates from the world of cryptocurrency and blockchain technology!
Image source: Shutterstock